As AI’s undeniable influence on technological advancement grows, so does the need to ensure that AI systems are trustworthy, ethical, and transparent. IBM, one of the leading forces in AI development, recognizes this imperative and has crafted several methods to ensure that its AI technologies uphold these values.
This blog will explore the key methods IBM uses to implement trustworthy AI solutions.
1. Ethical AI by Designed
The core of IBM’s AI development is a commitment to ethical principles, following the considerations and ethical limitations, and adhering to approved practices. IBM ensures that moral concerns are embedded into the AI design process right from the start. This involves processes according to a set of core ethical guidelines focused on fairness, transparency, and accountability.
- Fairness
Fairness and equal opportunities in AI mean ensuring that systems do not discriminate based on gender, race, age, or any other attributes that might lead to bias. IBM uses fairness controls to detect and mitigate bias in data sets and AI models. Adopting fairness measures enables them to ensure that the AI systems provide consistent and equitable outcomes for all.
For this very purpose, IBM’s AI Fairness 360 Toolkit is an open-source software library that helps developers identify and remove bias. This toolkit offers a variety of algorithms for AI models to produce fair results, which is essential in applications like recruitment, credit scoring, and healthcare.
- Transparency
Transparency is vital for building trust between AI systems and their users. IBM employs AI transparency by helping users understand how AI decisions are made. IBM’s tools, such as AI Explainability 360, help organizations achieve this by offering ways to interpret and explain AI models.
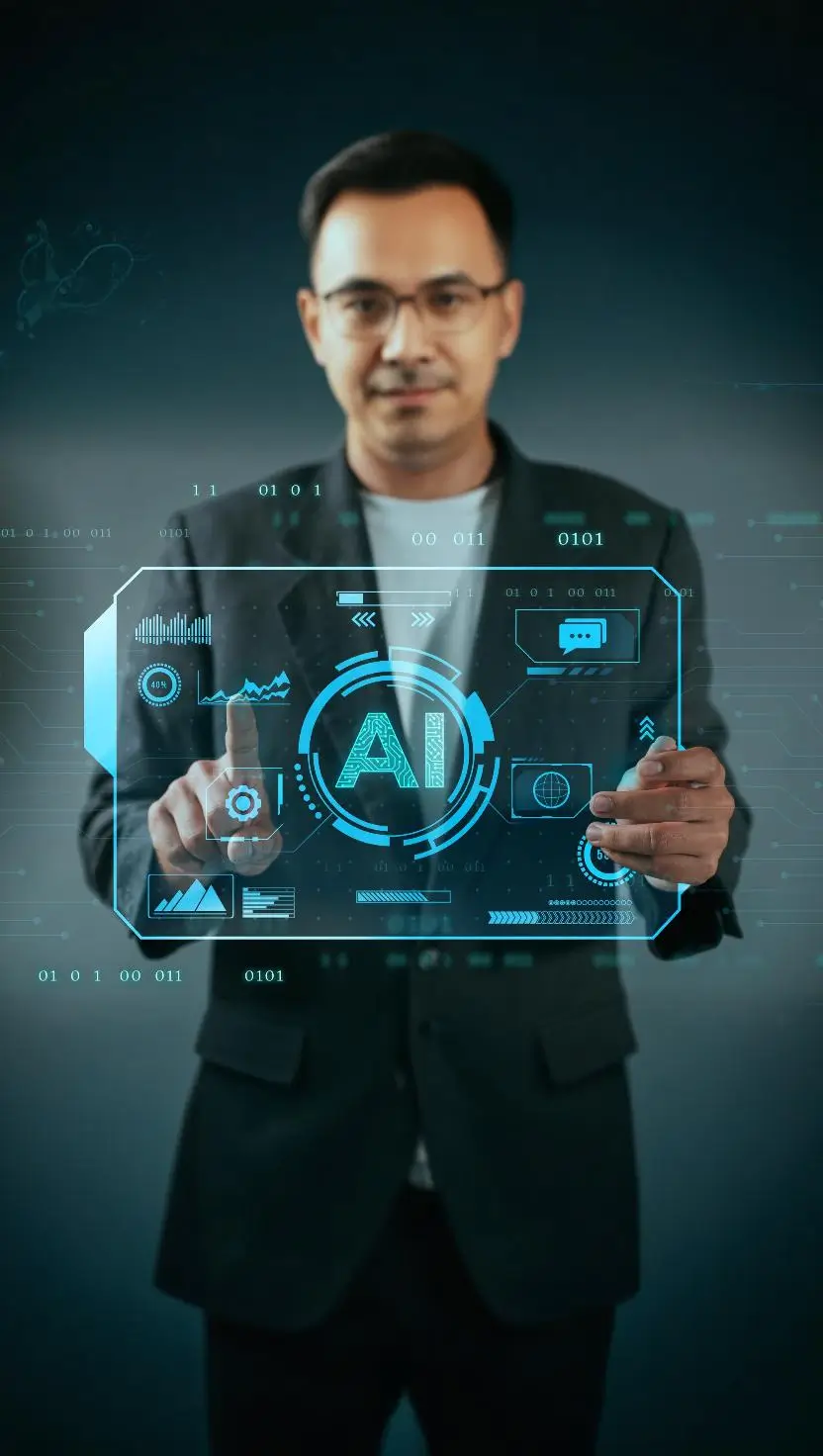
With transparent AI, users can see the decision-making process of AI systems, which is especially important in critical areas like healthcare diagnoses or legal verdicts. This transparency ensures users remain confident in the reliability of the systems.
- Accountability
IBM also upholds accountability in its AI solutions by making sure that developers and companies are responsible and accountable for the behavior of the AI systems they create. This makes it certain that there are mechanisms in place to address any unintended consequences or mistakes made by the system.
2. Solid Security and Privacy Measures
In the age where digital presence is mandatory, privacy and security are the most basic and genuine public concerns, and IBM takes these issues seriously when implementing AI solutions.
- Privacy-Preserving AI
IBM has developed steady methods to secure personal data when training AI models. One key technique is Federated Learning, which means AI systems learn from data without accessing it directly. Instead of centralizing data, IBM’s federated learning enables models to train on distributed data sources while keeping sensitive information local.
This approach is crucial for industries like healthcare, where patient confidentiality is paramount, or in financial services, where sensitive customer information must be protected.
- Data Anonymization
IBM also focuses on anonymizing data through techniques like differential privacy, which masks the data to protect individual identities while still providing accurate insights. This approach helps reduce the risk of exposing sensitive information, even in data-driven AI environments.
- Securing AI Systems
Security in AI is not just about protecting data but also ensuring that AI models themselves are secure from getting tampered with or receiving adversarial attacks. IBM uses techniques such as adversarial testing to stress-test AI systems, identifying potential vulnerabilities and weaknesses that could be exploited by malware. This is how they aim to create innovative AI models that are resilient to attacks.
3. AI Lifecycle Governance
IBM emphasizes governance throughout the entire lifecycle of AI development. This ensures that AI models are created, deployed, and maintained according to rigorous standards and guidelines.
- Model Monitoring
One of the key aspects of AI lifecycle governance is continuous model monitoring. IBM understands that AI models can degrade over time or become less accurate as data patterns change. To avoid this, they implement model monitoring systems that track the performance of AI models and alert teams if the models are no longer performing as expected.
This continuous monitoring ensures that AI models remain trustworthy even after they’ve been deployed into production.
- Feedback Loops
IBM incorporates feedback loops into AI systems, this is how models improve based on real-world user interactions. It’s particularly important in customer service applications, where AI systems must adapt to evolving customer needs and feedback.
These feedback mechanisms help IBM to refine AI models and ensure they remain accurate and effectively synced with trends over time.
4. Human-Centered AI
IBM’s trustworthy AI strategy includes creating AI systems that are designed for humans, which makes them relevant and popular. IBM believes that AI should enhance human capabilities rather than replace them. This human-centered approach ensures that AI solutions are developed with the end user in mind.
- AI for Business and Collaboration
IBM has developed AI systems that work smoothly with human teams, communicate, and collaborate rather than pose competition. IBM Watson is a leading AI platform designed to assist professionals in various industries, processing analytics, from healthcare to finance, and providing actionable insights and recommendations.
Watson is not built to replace the human workforce, instead, it enhances their decision-making abilities by processing vast amounts of data in ways that humans cannot, but always with a human in the loop to make the final call. This symbiotic relationship ensures that AI remains a tool for empowerment rather than displacement.
- Explainable AI for Trust
Explainability is central to human-centered AI, and for building explainable, trustworthy IBM focuses on making AI systems, which involves that the AI they build communicates their reasoning and decisions in a way that users can understand.
This is valuable for industries like healthcare or law enforcement, where stakeholders need to know why a particular recommendation or decision was made. IBM’s tools, like the aforementioned AI Explainability 360, help make AI more transparent, ensuring users can trust the systems they interact with.
5. Open AI Ecosystem
IBM has created an open ecosystem where developers, researchers, and businesses can access tools, libraries, and resources to build AI solutions responsibly. This admirable approach strengthens IBM’s commitment to trustworthy AI solutions and makes them stand out in the technological crowd.
- Open-Source Tools
By releasing open-source tools like AI Fairness 360 and Adversarial Robustness Toolbox, IBM has enabled the broader AI community to collaborate in solving the transparency challenges most AI models face. This open approach helps foster a collective effort toward building trustworthy AI systems across industries.
- Collaboration with Academia and Industry
IBM also collaborates with academic institutions, governments, and industry leaders to create ethical AI frameworks and regulations. This multi-dimensional approach ensures that AI technologies align with societal values and legal standards, further enhancing their trustworthiness.
6. Bias Mitigation and Auditing
Bias in AI is one of the most pressing concerns for developers and users alike. This is why IBM has taken proactive measures to address this issue through bias mitigation and auditing techniques.
- Bias Detection Tools
IBM’s bias detection tools analyze data sets for imbalances and anomalies that might lead to biased outcomes in AI models. This is especially crucial in applications like hiring, loan approvals, and criminal justice, where bias could lead to unfair treatment.
- Regular Auditing
IBM also conducts regular audits of AI systems to ensure they continue to meet ethical standards. These audits involve reviewing the data used for training AI models, as well as the outcomes produced by those models, to identify and mitigate any unintended bias.
7. Regulatory Compliance
In addition to ethical considerations, AI solutions need to comply with legal regulations and IBM does not oversee that. As AI adoption accelerates, so does the need for regulatory frameworks to guide its use.
- Adhering to Global Standards
IBM has active engagement with regulatory bodies and adherence to international standards for AI development, such as the OECD AI Principles and EU General Data Protection Regulation (GDPR). This is how they ensure that their AI solutions comply with data privacy laws and ethical guidelines.
- Promoting AI Regulation
IBM is also an advocate for responsible AI regulation, often calling for governments to establish clear guidelines and policies for AI use. This advocacy demonstrates IBM’s commitment to creating a balanced AI ecosystem where innovation thrives while societal values are respected.
Conclusion
Trustworthy AI solutions are not just a technical challenge; it’s an ethical responsibility that companies like IBM make their priority while establishing AI models. They integrate fairness, transparency, privacy, and collaboration into every step of AI development, IBM has positioned itself as a leader in the pursuit of ethical AI.
Through their innovative tools, commitment to open-source collaboration, and adherence to global standards, IBM paves the way for a future where AI not only accelerates progress but does so in a way that is fair, transparent, and aligned with human values.
FAQs
Q1: What are IBM’s 5 pillars of trustworthy AI?
IBM’s trustworthy AI framework is built on five key pillars to ensure its systems are responsible and reliable:
- Fairness: AI systems should provide unbiased and equitable results for all users.
- Explainability: Users should understand how AI systems reach their conclusions.
- Robustness: AI systems must be secure, resilient, and able to withstand adversarial attacks.
- Transparency: IBM ensures clarity in AI processes, making them understandable for users and stakeholders.
- Privacy: Personal data is safeguarded, and AI systems respect user privacy through secure practices like federated learning.
Q2: What are the three main principles IBM follows for ethical AI?
IBM adheres to three core ethical AI principles to guide its AI development and use:
- Fairness: Mitigating bias to ensure AI delivers fair and unbiased results.
- Transparency: Clear and open communication about how AI systems work and make decisions.
- Accountability: Ensuring that developers and organizations remain responsible for AI systems and their impact on users.
Q3: What is the AI system developed by IBM?
IBM’s flagship AI system is Watson, an advanced AI platform that uses machine learning and natural language processing. Watson is designed for a wide range of applications, such as:
- Analyzing large datasets to uncover insights.
- Enhancing customer service through chatbots and automation.
- Assisting industries like healthcare, finance, and retail with smart decision-making.
Q4: What are the goals of the IBM AI system?
The goals of IBM’s AI systems, including Watson, are to:
- Enhance decision-making: Provide data-driven insights to improve accuracy and outcomes.
- Automate tasks: Streamline processes and reduce the manual workload in various industries.
- Improve customer experiences: Offer personalized, efficient, and high-quality interactions.
- Drive innovation: Empower businesses to solve complex problems using cutting-edge AI technologies.
Q5: Which statement best defines IBM’s AI governance?
IBM’s AI governance ensures responsible development, deployment, and usage of AI technologies by:
- Establishing ethical guidelines and best practices.
- Continuously monitoring AI systems to ensure fairness, transparency, and compliance with regulations.
- Ensuring that AI systems align with societal values and legal frameworks while protecting user privacy.